Why Kado
By honing in on ML, your vision becomes narrow
We show you how to look broad and help bring balance.
By ignoring problem definition, you miss out on application
We help take blinders off and better interact with stakeholders.
By blending methods together, you expand your repertoire
We guide you on Solution Architecture in an experimental setting
By mastering the experimental mindset, you better prep for AI
We mitigate your chances of getting blindsided
Write your awesome label here.
What are Hero Methods?
While Machine Learning is amazing, it's not the only method to solve problems in AI.
We introduce you to the full palette and how to apply them.
We introduce you to the full palette and how to apply them.
Complex in nature
Problems solved by Hero Methods - Hero Problems - are not effectively modelled by simple rules, as you find in most software.
List of Methods
Machine Learning, Optimization, Simulation, Knowledge Representation, Search/Planning, Dealing with Uncertainty, High Performance Computing.
Brute Force is infeasible
To solve complex problems, we need concise reliable methods that abstract & generalize the underlying patterns with efficient compute that can process formal representations.
"Good enough" is good enough
Complex problems may not have exact answers. The process to solve them is beset with uncertainty and may yield different results every time. Approximate, "rule of thumb" solutions are sufficient.
Problem Definition is your weapon.
Better understand your domain, relate to your audience and lead to consensus.
Ignore it at your peril. We show you how to do it right!
Ignore it at your peril. We show you how to do it right!
#1 Hero vs. Non Hero
Unlike classic software builds, for problems in AI, the solution is not always known. Much experimentation is needed to surface the answer.
#2 Common Ground
Regardless of which, you are never going to solve it right, if you don't know what you're solving. Problem Definition is the first thing you'll need to do.
#3 Maven of Modelling
Problem, not solution. You'll learn about it from stakeholders. You then decompose it into sub-problems - in different ways. The end goal - Modelling.
#4 Shepherd Stakeholders
Then, teach stakeholders the many dimensions of an AI problem. Show them how and why you decomposed yours, and get their feedback. Earn buy-in & consensus.
Architecture in AI is Experimental
Write your awesome label here.
Why the long view matters
We show you what you can do now to prepare.
1. AGI vs Machine Learning
Those in the AGI community will tell you AI will be here sooner than you think. They treat AI as something more than Machine Learning. Unlike the latter, most AI reside in dark sites around the world. Government, military, protected. Regardless of what form it takes, when it arrives, it's going to change everything!
2. Foundations of AGI
Regardless of where AI resides, Hero Methods - Machine Learning, Optimization, Simulation, Knowledge Representation, Search/Planning, Dealing with Uncertainty & High Performance Computing - will form its bases.
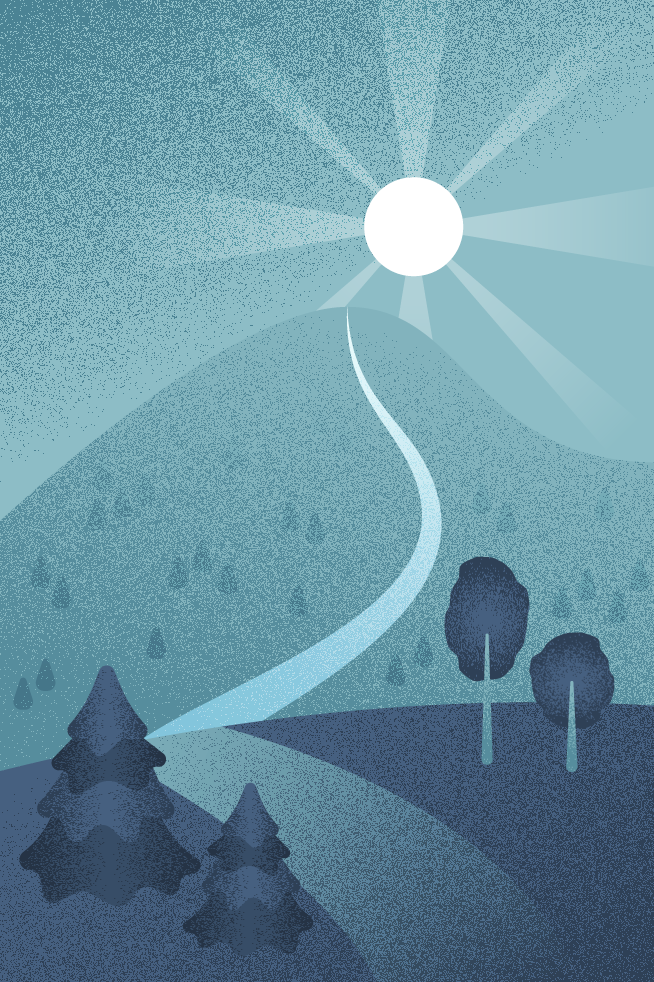
3. Present and Future
As a result, AI is also experimental in nature. Learn how to experiment with Hero Methods now and you’ll have gained the ability to adopt/adapt AI when it arrives. Thankfully, there is a rich abundance in use cases and applications today - no need to look far. If you wait till AI is here, it will be too late.
4. Looking ahead
At Kado, we are forward thinkers. In order to get ready for the road ahead, we are betting our present on Hero Methods. Experimenting is part of our DNA and we can show you how to make it a part of yours.
Love free content that is cool?
Thank you!