A compelling Paradigm in AI: Generative Design
Oct 17, 2022 | Jagannath Rajagopal | 3 min read
Generative Design (GD) is a paradigm in which traditional design is carried out in collaboration with a computer system. Unlike a delegate system running models proposed by the user, the machine acts as part of a co-creative process where it suggests design alternatives in collaboration with the user. These are formulated by simulating, optimizing, and estimating scenarios that allow the exploration of the solution space.
The system needs to be smart enough to expand the proposed paths suggested by the designer and release her from the burden of exhaustive specification. It is not automatic design, it is (heavily) assisted-design performed with an autonomous agent. The agent may need to interface with one or more existing systems to pull design options and detailed specs together. In some domains, design is managed in existing systems like CAD. In those cases, the agent may create the design with all its details in those systems. In this sense, the agent does not act as the system of record for the design; instead it acts as a middle-man between the designer and design system.
This approach is especially useful where an organization wishes to take GD incrementally in steps. Organizations today have processes and systems built around manual design; an approach that allows re-use of existing infrastructure will make the process of adapting to GD much easier.
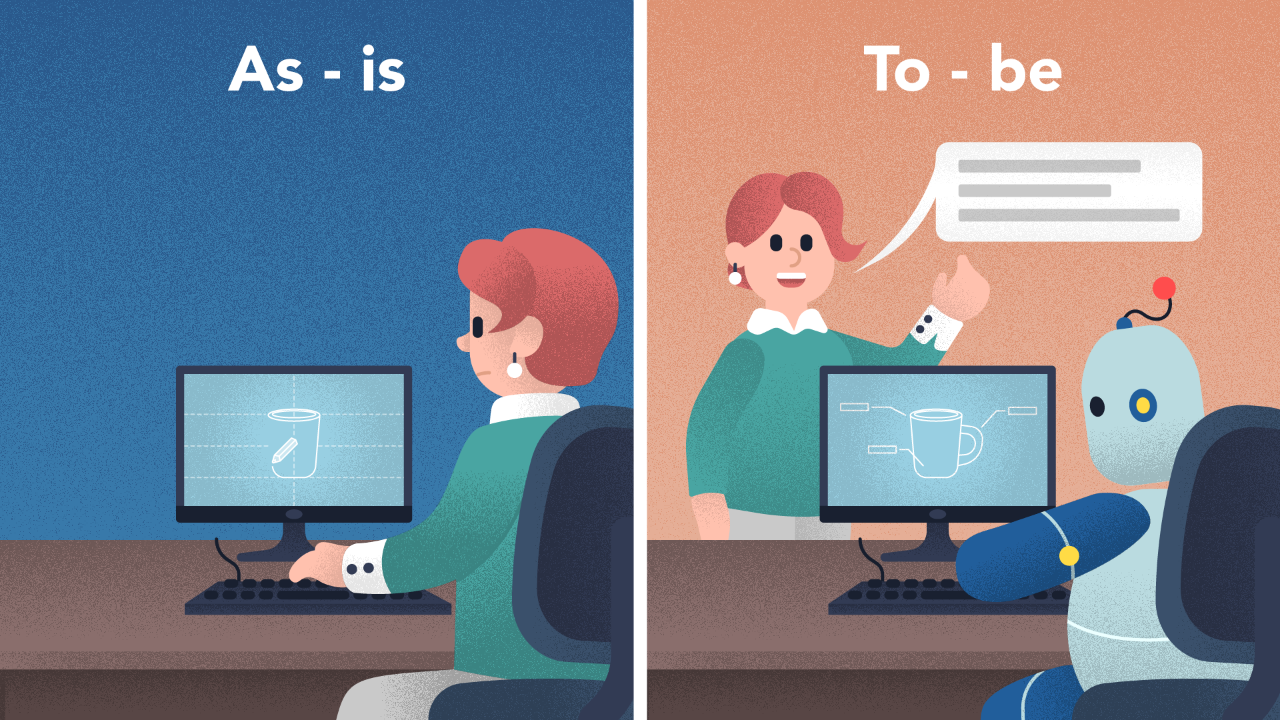
I’ve created a LOT of resources on topics like these. Here’s my course on Design Thinking for Hero Methods. Here’s my YouTube channel with previews of course videos. Here’s my website; navigate through the courses to find free previews & pdfs.
- - -
This paradigm applies to many different domains and fields — in engineering, GD may be applied to design of various types of structures and machinery. Traditional engineering design involves methods like computer aided design, finite element analysis, computational fluid dynamics etc which require a designer to manually select and imagine various design specifications. With GD, a computational system takes on the onus of coming up with the detailed design freeing up the designer to suggest high level design objectives and choose from amongst design options put forth by the machine.
GD may also be used in drug design and computational biology to formulate complex molecular structures to tailor drugs that exhibit certain biochemical behaviours. With traditional approaches, it was left to research teams to imagine complex structures. Over time, we have now gathered a vast wealth of data on chemistry and molecular interactions, which may then be utilized by a computational system to imagine and co-create various structures with drug designers towards certain objectives. Better drugs = lesser side effects, better cures, increased revenues/reputation etc.
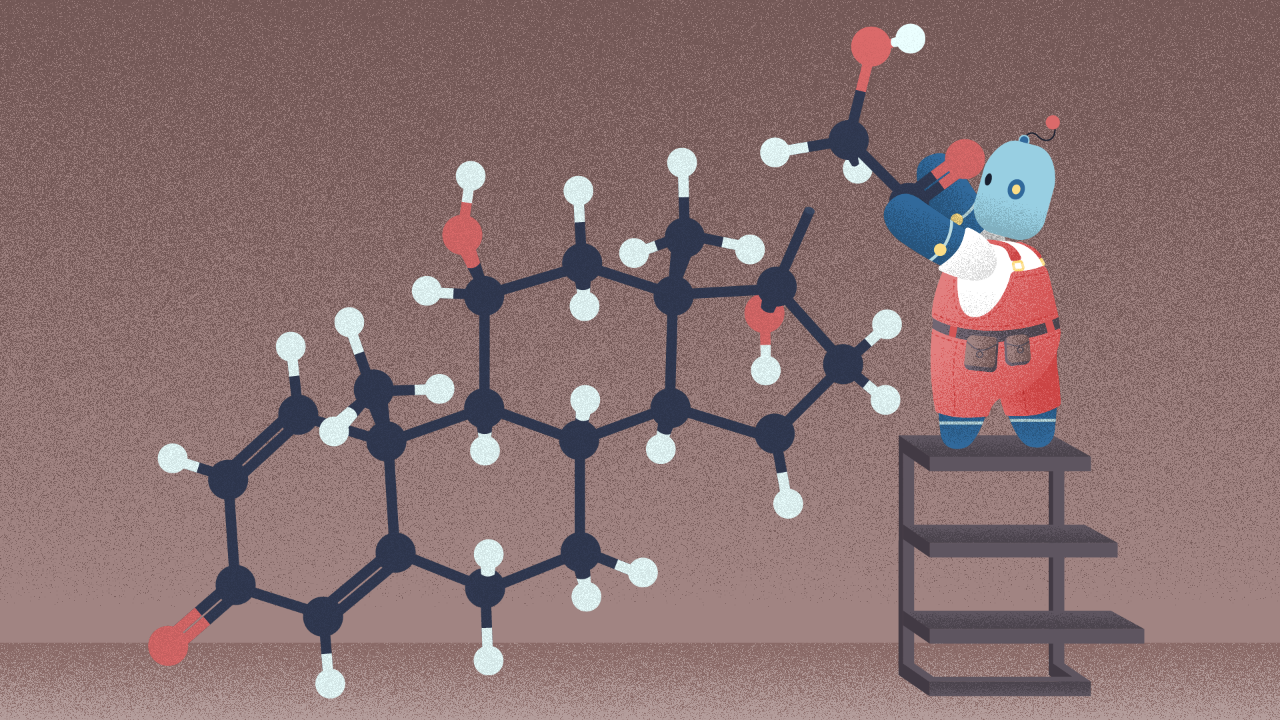
There are several applications of GD. One interesting area is the design of complex social and economic systems based on behaviours of individuals or groups within them. In a financial market, you will find participants that play various roles — HFTs, money managers, market-makers, hedge funds, etc — and each of these come to the market with a slightly different objective and need. If you simulate individuals in a market interacting with one another, that can be scaled up to the market level. This can be done in different ways, and GD may be used in this process to accomplish certain objectives like profit maximization, visibility into demand/supply or calibrated levels of access. The challenges here would be managing various types of uncertainty and randomness inherent in the markets.
At Kado, we love Generative Design. The paradigm is broad & flexible, and allows for more than one Hero Method to work together.
This is how we see the world
Machine Learning is King, but of narrow territory. Hero Methods do things that ML cannot. Taken together, not only do they help solve complex problems, they also lay the doorway to AI.
Get in touch
-
Milton, ON L9T 6T2, Canada
-
help@kado.ai
-
+1 416 619 0517
Copyright © 2025
Super!
An email is on it's way.
Super!
An email is on it's way.